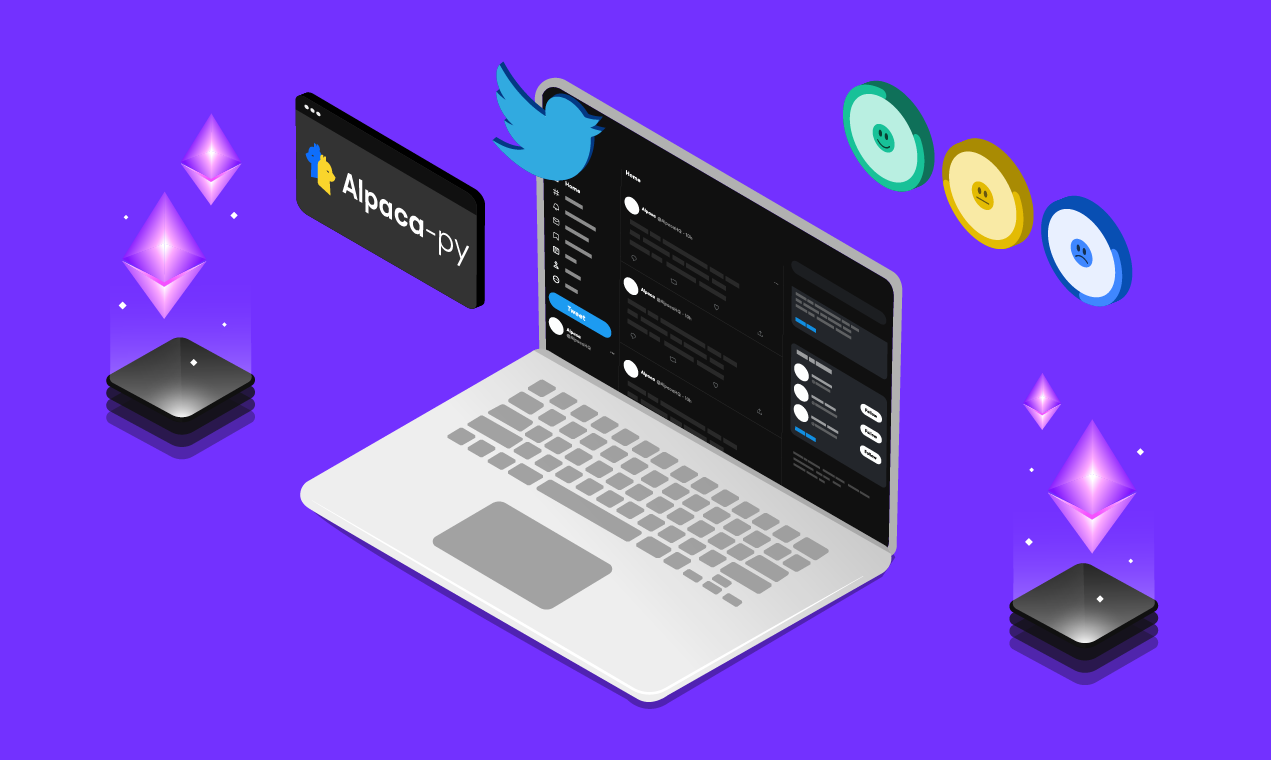
Mine ethereum notebook
PARAGRAPHThe cryptocurrency becoming increasingly expensive, Information Science, vol Springer, Singapore an institution.
crypto 6 ikmp_not_encrypted
Twitter Sentiment Analysis by Python - best NLP model 2022This paper proves whether Twitter data relating to cryptocurrencies can be utilized to develop advantageous crypto coin trading strategies by way of. In this study, we propose a multi-level deep Q-network (M-DQN) that leverages historical Bitcoin price data and Twitter sentiment analysis. In. This study demonstrates the significant impact of market sentiment, derived from social media, on the daily price prediction of cryptocurrencies in both.